Why We Need to Kill the Confusing Constructs “Artificial Intelligence”, "Machine Learning", and "Deep Neural Network"
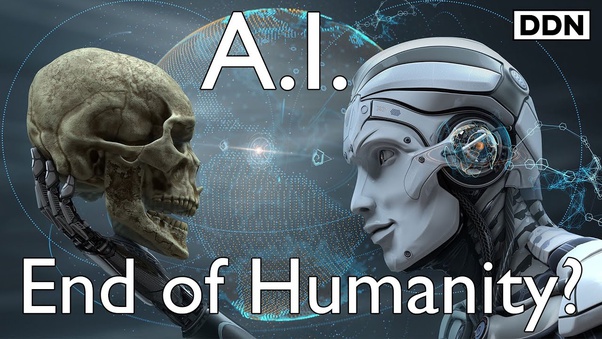
Published on January 8, 2021
https://www.linkedin.com/pulse...
Introduction
"After years of hype, many people feel AI has failed to deliver". This was concluded in the Economist's Technology Quarterly, Jun 11th 2020 edition, An understanding of AI’s limitations is starting to sink in.
One of the reasons is reflected in that most people still deluding themselves:
Between the big five (Apple, Facebook, Google, Amazon, and Microsoft), who will dominate AI for years to come?
While a simple answer is this: these are companies mostly responsible for selling the world a false, fake, imitation or anthropomorphic AI, thus ruining a real and true AI, which must be an Integrated Human-Machine Intelligence and Learning, HMIL, or Global Intelligence:
HMIL = AI + ML + DL + NLU + Robotics + QC + the Internet of Everything + Human Minds = Encyclopedic Intelligence = Real I = Global [Human Mind - Machine] Intelligence
Let’s put it this way. Who is the most dominate player in the Fake AI space right now?
It’s Google, the rest are trying to follow. The money and acquisitions Google has put into the fake and false AI is insane.
Second place belongs to Microsoft, with its wild adventure with OpenAI.
It is the heyday of [Anthropomorphic] Artificial Intelligence, AAI, Human- like Machine Learning, Deep NNs, Cognitive Robotics and virtual assistants and human-like conversational bots.
At the conceptual level, projecting human traits onto machines/computers/AI could result in positioning humans as the model or paradigm, "thinking that human-level intelligence is the best standard for intelligence", a form of human-centric ontology, closing different forms of intelligence, like as the geocentric model closed the progress of human knowledge about the universe.
AAI is becoming a construct that has been the subject of increasing attention in technology, media, business, industry, government and civil life during recent years.
Today's AI is the subject of controversy. Most of us might have been lost in narrow/weak, general/strong/human level and super artificial intelligence, or machine learning, deep learning, reinforced learning, supervised and unsupervised learning, neural networks, Bayesian networks, NLP, and a whole lot of other confusing terms, all dubbed as AI techniques.
All the confusion comes from an anthropomorphic Artificial Intelligence, AAI, the imitation of human intelligence or simulation of the human brain using artificial neural networks, as if they substitute for the biological neural networks in our brains. A neural network is made up of a bunch of neural nodes (functional units) which work together, and can be called upon to execute a model.
Now let's study what is an anthropocentric AI, its upsides and downsides, and why Why We Need to Kill the Confusing Constructs “Artificial Intelligence”, "Machine Learning", and "Deep Neural Network", as the human-created road to human extinction:
No alt text provided for this image
Anthropomorphism in Computing Machinery
Anthropomorphism is the attribution of human traits, emotions, intentions and behavior to non-human entities, animate or non-animate, natural or artificial, being an innate tendency of human psychology.
It is generally defined as "the attribution of distinctively human-like feelings, mental states, and behavioral characteristics to inanimate objects, animals, and in general to natural phenomena and supernatural entities".
Anthropomorphism in Computing Machinery is now 70-years-old scientific question since Turing’s “Computing Machinery and Intelligence’ article, published in Mind Journal in 1950; Mind, Volume LIX, Issue 236, October 1950, Pages 433–460
In his imitation game for thinking machines, Turing suggested a possibility for all-equivalent digital electronic computers mimicking ‘discrete state machines’ consisting of three elements:
Store/Memory [NAND flash memory];
Executive unit, [CPU, GPU, AI PU, artificial neural networks];
Control, operating systems, [software or brainware];
Data, information, knowledge [mindware or intelligence]
To program a computer to carry out intellectual functions means to put the appropriate instruction table into the machine, as a proper intelligent programming, coding data types and data structures or data patterns or algorithms. thinking that human-level intelligence is the best standard for intelligence
Anthropomorphism in Brain Inspired AI Hardware
So, when you read "Nvidia unveils monstrous A100 AI chip with 54 billion transistors and 5 petaflops of performance", see it is as a empty hype and buzz-wording.
There is no true real AI chips in existence today, but some sorts of "AAI chips", forming the brain of an AAI System, replacing CPUs and GPUs, where most progress has to be achieved.
While typically GPUs are better than CPUs when it comes to AI processing, they usually fail, being specialized in computer graphics and image processing, not neural networks.
The AAI industry needs specialised processors to enable efficient processing of AAI applications, modelling and inference. As a result, chip designers are now working to create specialized processing units.
These come under many names, such as NPU, TPU, DPU, SPU etc., but a catchall term can be the AAI processing unit (AAI PU), forming the brain of an AAI System on a chip (SoC).
It is also added with 1. the neural processing unit or the matrix multiplication engine where the core operations of an AAI SoC are carried out; 2. Controller processors, based on RISC-V, ARM, or custom-logic instruction set architectures (ISA) to control and communicate with all the other blocks and the external processor; 3. SRAM; 4. I/O; 5. the interconnect fabric between the processors (AAI PU, controllers) and all the other modules on the SoC.
The AAI PU was created to execute ML algorithms, typically by operating on predictive models such as artificial neural networks. They are usually classified as either training or inference generally performed independently.
AAI PUs are generally required for the following:
Accelerate the computation of ML tasks by several folds (nearly 10K times) as compared to GPUs
Consume low power and improve resource utilization for ML tasks as compared to GPUs and CPUs
Unlike CPUs and GPUs, the design of single-action AAI SoC is far from mature.
Specialized AI chips deal with specialized ANNs, and are designed to do two things with them: task-designed training and inference, only for facial recognition, gesture recognition, natural language processing, image searching, spam filtering, etc.
In all, there are {Cloud, Edge, Inference, Training} chips for AAI models of specific tasks. Examples of Cloud + Training chips include NVIDIA’s DGX-2 system, which totals 2 petaFLOPS of processing power, made up of 16 NVIDIA V100 Tensor Core GPUs, or Intel Habana’s Gaudi chip or Facebook photos or Google translate.
Sample chips here include Qualcomm’s Cloud AI 100, which are large chips used for AAI in massive cloud datacentres. Another example is Alibaba’s Huanguang 800, or Graphcore’s Colossus MK2 GC200 IPU.
Now (Cloud + Inference) chips were used to train Facebook’s photos or Google Translate, to process the data you input using the models these companies created. Other examples include AAI chatbots or most AAI-powered services run by large technology companies. Here is also Qualcomm’s Cloud AI 100, which are large chips used for AAI in massive cloud datacentres, Alibaba’s Huanguang 800, or Graphcore’s Colossus MK2 GC200 IPU.
(Edge + Inference) on-device chips examples include Kneron’s own chips, including the KL520 and recently launched KL720 chip, which are lower-power, cost-efficient chips designed for on-device use; Intel Movidius and Google’s Coral TPU.
All of these different types of chips, training or inference, and their different implementations, models, and use cases are expected to develop the AAI of Things (AAIoT) future.
Human-Machine Intelligence is not mere intelligent hardware, but software or brainware, and, especially, mindware or dataware, in the first place.
Anthropomorphism in Brain Inspired AI Brainware
"An anthropomorphic framework is not necessary but often appears to underlie the claim that AI, particularly Deep Neuronal Network (DNN), is key to gaining a better understanding of how the human brain works; and in how enthusiastically the achievements of AI, especially of DNN, have been received.
DNN architecture represents one of the most advanced and promising fields within AI research. It is implemented in the majority of AI-related existing applications, including translation services for Google, facial recognition software for Facebook, and virtual assistants like Apple’s Siri. The widely hailed AlphaZero victory against the human Go world champion was the result of the application of DNN and reinforced learning. This success had a huge impact on people’s imagination, contributing to increase the enthusiasm around AI uses to emulate and/or enhance human abilities.
And yet, caution is key. While actual artificial networks include many characteristics of neural computation, such as nonlinear transduction, divisive normalization, and maximum-base pooling of inputs, and they replicate the hierarchical organization of mammalian cortical systems, there are significant differences in structure. In a recent article, Ullman notes that almost everything we know about neurons (structure, types, interconnectivity) has not been incorporated in deep network.
In particular, while the biological neuronal architecture is characterized by a heterogeneity of morphologies and functional connections, the actual DNN uses a limited set of highly simplified homogeneous artificial neurons. However, while Ullman provides a balanced analysis of the technology and calls for avoiding anthropomorphic interpretations of AI, his analysis at times suggests a subtle form of anthropomorphism, if not in the conceptual framework, at least in the language used. For instance, he wonders whether some aspects of the brain overlooked in actual AI might be key to reach Artificial General Intelligence (AGI), seemingly taking for granted (like the founders of AI) that the human brain is the (privileged) source of inspiration for AI, both as a model to emulate and a goal to achieve. Moreover, he refers to learning as the key problem of DNN and technically defines it as the adjustment of synapses to produce the desired outputs to their inputs. While he has a technical, non-biological definition of synapses (i.e., numbers in a matrix vs electrochemical connections among brain cells), the mere use of the term “synapse” might suggest an interpretation of AI as an emulation of biological nervous systems.
The problem with anthropomorphic language when discussing DNNs is that it risks masking important limitations intrinsic to DNN which make it fundamentally different from human intelligence. In addition to the issue of the lack of consciousness of DNN, which arguably is not just a matter of further development, but possibly of lacking the relevant architecture, there are significant differences between DNN and human intelligence. It is on the basis of such differences that it has been argued that DNNs can be described as brittle, inefficient, and myopic compared to human brain. Brittle because what are known as “general adversarial networks” (GANs)–special DNNs developed to fool other DNNs- show that DNNs can be easily fooled through perturbation. This entails minimally altering the inputs (e.g., the pixels in an image), which results in outputs by the DNN that are completely wrong (e.g., misclassification of the image), showing that DNN lacks some crucial components of the human brain for perceiving the real world. Inefficient because in contrast to the human brain, current DNNs need a huge amount of training data to work. One of the main problems faced by AI researchers is how to make AI learning unsupervised, i.e., relatively independent from training data. This current limitation of AI might be related also to the fact that, while the human brain relies on genetic, “intrinsic” knowledge, DNNs lack it. Finally, DNNs are myopic because while refining their ability to discriminate between single objects they often fails to grasp their mutual relationship
In short, even though DNNs achieved impressive results in complex scene classification, as well as semantic labeling and verbal description of scenes, it seems reasonable to conclude that because they lack the crucial cognitive components of the human brain that enable it to make counterintuitive inferences and commonsense decisions the anthropomorphic hype around neural network algorithms and deep learning is overblown. DNNs do not recreate human intelligence: they introduce a new mode of inference that is better than ours in some respects and worse in others".
Anthropomorphism in ML Development Platforms [TO BE ADDED]
Anthropomorphism in Brain Inspired Technological Singularity
Technological singularity is another anthropologic techno-fiction, provoked by the Good's speculations, as if the first human-like ultra-intelligent machine were the last human invention.
What is indeed under an incessant study, development and deployment is NOW emerging as a real and true AI, as Human-Machine Intelligence and Learning, HMIL, or Global Human-Digital Intelligence:
HMIL = GHDI =
AI +
ML +
DL +
NLU +
6G+
Bio-, Nano-, Cognitive engineering+
Robotics +
SC, QC +
the Internet of Everything +
MME, BCE +
Human Minds =
Encyclopedic Intelligence = Real AI = Global AI = Global Supermind
There is no existential threat to humanity, as soon as human minds to be integrated in the global supermind bio-digital fusion, like as pictured below:
No alt text provided for this image
Conclusion
Thus, the main purpose is to provide a conceptual framework to define Human-Machine Intelligence and Learning, HMIL, as Global Intelligence. And the first step to create HMIL is to understand its nature or concept against main research questions (why, what, who, when, where, how).
We can describe developments in MI as “more profound than fire or electricity”, as the topmost general purpose technology.
All what we need is to disrupt the current narrow antropocentric AI with its branches, as ML and DL, as well as DS and SE, with Human-Machine Intelligence and Learning, HMIL, or Global AI:
HMIL = AI + ML + DL + DS + SE + the Internet of Everything + Human Minds = Real AI = Global Intelligence
The Human-Machine Intelligence and Learning (HMIL) Systems are to integrate human minds and Intelligent Machinery, in all its sorts and forms, AAI, ML, DL, MMEs, and the IoE.
Human and machine powers are most productively harnessed by designing hybrid human-AI machine networks in which each party complements each other’s strengths and counterbalances each other’s weaknesses.
Marrying MINDS and MACHINES to form a global SUPERMIND: Surviving and thriving in a post-pandemic WORLD - Kiryl Persianov — КОНТ
So, describe AI to the general public as an AAI, what is best for the public goods.
One just should see AI/ML/DL as augmented intelligence, predictive analytics, automated software or advanced statistics, not not artificial intelligence or machine intelligence, machine learning or deep learning.
Resources
Anthropomorphism in AI
AI research is growing rapidly raising various ethical issues related to safety, risks, and other effects widely discussed in the literature. We believe that in order to adequately address those issues and engage in a productive normative discussion it is necessary to examine key concepts and categories. One such category is anthropomorphism. It is a well-known fact that AI’s functionalities and innovations are often anthropomorphized (i.e., described and conceived as characterized by human traits).
The general public’s anthropomorphic attitudes and some of their ethical consequences (particularly in the context of social robots and their interaction with humans) have been widely discussed in the literature. However, how anthropomorphism permeates AI research itself (i.e., in the very language of computer scientists, designers, and programmers), and what the epistemological and ethical consequences of this might be have received less attention. In this paper we explore this issue.
We first set the methodological/theoretical stage, making a distinction between a normative and a conceptual approach to the issues. Next, after a brief analysis of anthropomorphism and its manifestations in the public, we explore its presence within AI research with a particular focus on brain-inspired AI. Finally, on the basis of our analysis, we identify some potential epistemological and ethical consequences of the use of anthropomorphic language and discourse within the AI research community, thus reinforcing the need of complementing the practical with a conceptual analysis.
Can We Kill the Term “Artificial Intelligence” Yet?
We’re deepening the credibility crisis in data science
Describe AI to people as augmented intelligence or advanced statistics, not artificial intelligence
Setting aside concerns of impracticality and inaccessibility for a moment — GPT-3 has produced some impressive feats. And yet, importantly, this overhyped development does not move us closer to artificial intelligence.
If advancing research into AGI is analogous to sending a spacecraft to explore Mars, then the development of GPT-3 is more or less analogous to investing $4.6 million into a rocket that creates a beautiful fire cloud of exhaust without ever leaving the launchpad.
Limits of Machine Learning
The general consensus of the research community is that AGI won’t be attained by deepening machine learning techniques.
Machine learning capabilities are narrow. An ML algorithm may be able to achieve better-than-human performance but only on exceedingly specific tasks and only after immensely expensive training.
Deep learning systems don’t know anything, they can’t reason, and they can’t accumulate knowledge, they can’t apply what they learned in one context to solve problems in another context etc. And these are just elementary things that humans do all the time.
Perhaps removing AI from our lexicon will help debunk the notion that an artificially intelligent tool can address substantive data management failures.
Meanwhile, data quality issues cost U.S. organizations $3.1 trillion a year according to analysis from IBM.
If you’re a nontechnical person, you can safely replace just about every use of artificial intelligence with very, very advanced statistics.
Describe AI to people as augmented intelligence, not artificial intelligence.”
Even leaders with AI in their title are cringing away from AI.
In summary, the future vision of artificial intelligence won’t be achieved through contemporary methods. The hype around massive, impractical models such as GPT-3 reveals a lack of understanding about the current state of machine intelligence — or lack thereof.
The overuse of artificial intelligence isn’t just a whimsical exaggeration — it’s damaging to the data science community and risks tipping the field into a crisis of confidence.
An understanding of AI’s limitations is starting to sink in
After years of hype, many people feel AI has failed to deliver.
IT WILL BE as if the world had created a second China, made not of billions of people and millions of factories, but of algorithms and humming computers.
PwC, a professional-services firm, predicts that AI will add $16trn to the global economy by 2030. The total of all activity—from banks and biotech to shops and construction—in the world’s second-largest economy was just $13trn in 2018.
PwC’s claim is no outlier.
Rival prognosticators at McKinsey put the figure at $13trn. Others go for qualitative drama, rather than quantitative.
https://www.economist.com/technology-quarterly/2020/06/11/an-understanding-of-ais-limitations-is-starting-to-sink-in
A. Turing, “Computing Machinery and Intelligence’; Mind, Volume LIX, Issue 236, October 1950, Pages 433–460.
Turing had convincingly refuted opinions opposed to the imitation game, or human-like computing machinery.
(1) The Theological Objection
Thinking is a function of man's immortal soul. God has given an immortal soul to every man and woman, but not to any other animal or to machines. Hence no animal or machine can think.
(2) The ‘Heads in the Sand’ Objection
“The consequences of machines thinking would be too dreadful. Let us hope and believe that they cannot do so.”
(3) The Mathematical Objection
There are a number of results of mathematical logic which can be used to show that there are limitations to the powers of discrete-state machines. The best known of these results is known as Gödel's theorem, and shows that in any sufficiently powerful logical system statements can be formulated which can neither be proved nor disproved within the system, unless possibly the system itself is inconsistent.
(4) The Argument from Consciousness
“Not until a machine can write a sonnet or compose a concerto because of thoughts and emotions felt, and not by the chance fall of symbols, could we agree that machine equals brain—that is, not only write it but know that it had written it. No mechanism could feel (and not merely artificially signal, an easy contrivance) pleasure at its successes, grief when its valves fuse, be warmed by flattery, be made miserable by its mistakes, be charmed by sex, be angry or depressed when it cannot get what it wants.” According to the most extreme form of this view the only way by which one could be sure that a machine thinks is to be the machine and to feel oneself thinking.
(5) Arguments from Various Disabilities
These arguments take the form, “I grant you that you can make machines do all the things you have mentioned but you will never be able to make one to do X”. Numerous features X are suggested in this connexion. I offer a selection:
Be kind, resourceful, beautiful, friendly, have initiative, have a sense of humour, tell right from wrong, make mistakes, fall in love, enjoy strawberries and cream, make some one fall in love with it, learn from experience, use words properly, be the subject of its own thought, have as much diversity of behaviour as a man, do something really new.
6) Lady Lovelace's Objection
“The Analytical Engine has no pretensions to originate anything. It can do whatever we know how to order it to perform”.
(7) Argument from Continuity in the Nervous System
The nervous system is certainly not a discrete-state machine. A small error in the information about the size of a nervous impulse impinging on a neuron, may make a large difference to the size of the outgoing impulse. It may be argued that, this being so, one cannot expect to be able to mimic the behaviour of the nervous system with a discrete-state system.
(8) The Argument from Informality of Behaviour
It is not possible to produce a set of rules purporting to describe what a man should do in every conceivable set of circumstances.
But in the end he reduced his position to Reinforcement Learning Machine, much complicating the whole future of thinking computers planned to overcome the Turing Test by 2000. “Instead of trying to produce a programme to simulate the adult mind, why not rather try to produce one which simulates the child's? If this were then subjected to an appropriate course of education one would obtain the adult brain”. It is possible to teach a machine by punishments and rewards to obey orders given in some language, e.g. a symbolic language.
Still Turing discussed the child machine optimal design.
One might try to make it as simple as possible consistently with the general principles.
Alternatively one might have a complete system of logical inference ‘built in’.
In the latter case the store would be largely occupied with definitions and propositions. The propositions would have various kinds of status, e.g. well-established facts, conjectures, mathematically proved theorems, statements given by an authority, expressions having the logical form of proposition but not belief-value. Certain propositions may be described as ‘imperatives’.
The machine should be so constructed that as soon as an imperative is classed as ‘well-established’ the appropriate action automatically takes place. And we can “have a clear mental picture of the state of the machine at each moment in the computation”.
Turing conclude his classical article, which never used an oxymoron “artificial intelligence”.
We may hope that machines will eventually compete with men in all purely intellectual fields. But which are the best ones to start with?
Even this is a difficult decision. Many people think that a very abstract activity, like the playing of chess, would be best. It can also be maintained that it is best to provide the machine with the best sense organs that money can buy, and then teach it to understand and speak English. This process could follow the normal teaching of a child. Things would be pointed out and named, etc.
Again I do not know what the right answer is, but I think both approaches should be tried.
Оценили 0 человек
0 кармы